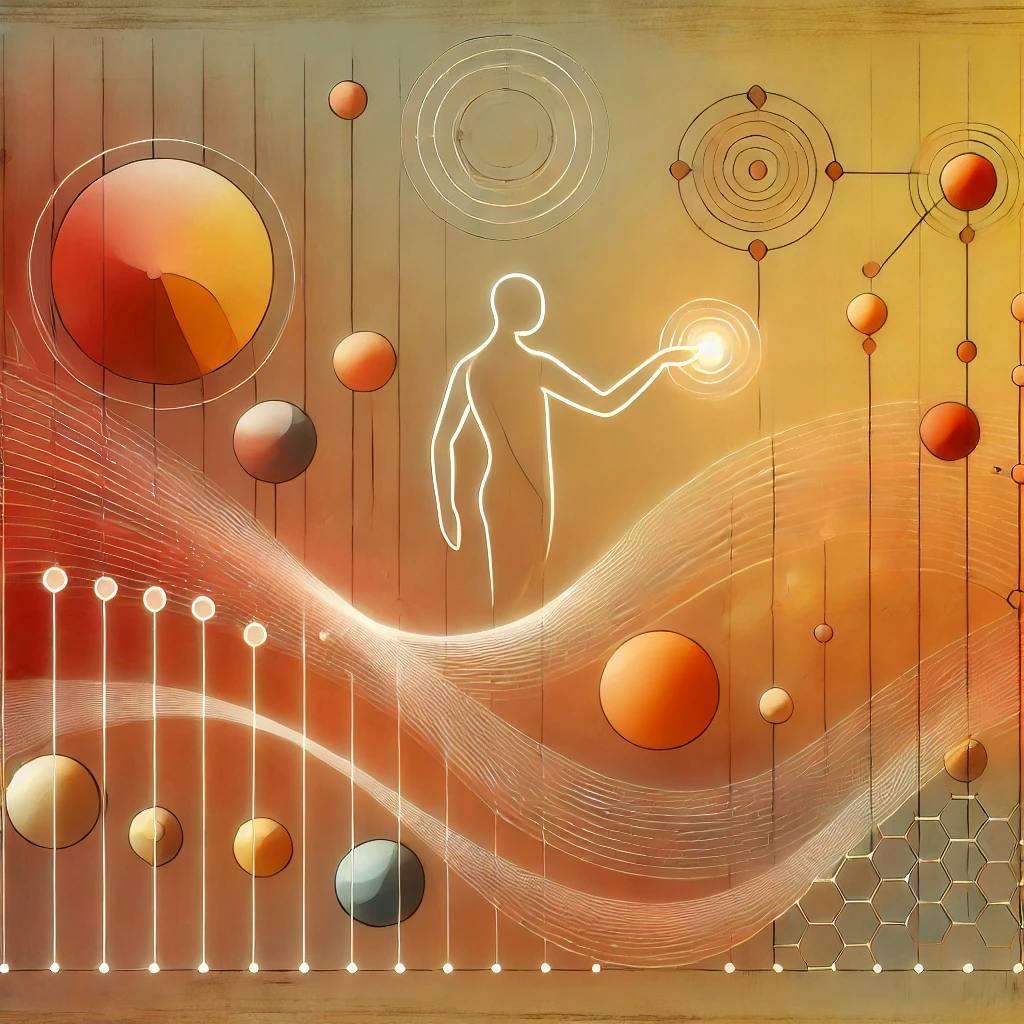
Systems of Intelligence
Optimizing the way we work
On December 1, 1913, Henry Ford implemented the first moving assembly line for the mass production of a whole car. This dramatically reduced a process of 84 different steps that previously required over 12 hours of mostly manual labour for completion to just 93 minutes.
Ford’s revolution is just one of many instances where economic growth and technological advancement has transformed the nature of work. Already, as we move into the age of AI and the fourth industrial revolution, a new approach is emerging: Systems of Intelligence.
What are Systems of Intelligence?
Similar to the moving assembly line, Systems of Intelligence (SOI) use new technology to accelerate full, traditional workflows—but for data, rather than physical manufacturing. They are a gateway to digital transformation.
More specifically, our CEO Siv Kailasapathy describes:
A System of Intelligence is an end-to-end solution designed to execute a series of workflows typically spanning several disciplines for large volumes of data. It’s powered by human-in-the-loop machine learning and knowledge graphs to achieve objectives in a significantly shorter timeframe.
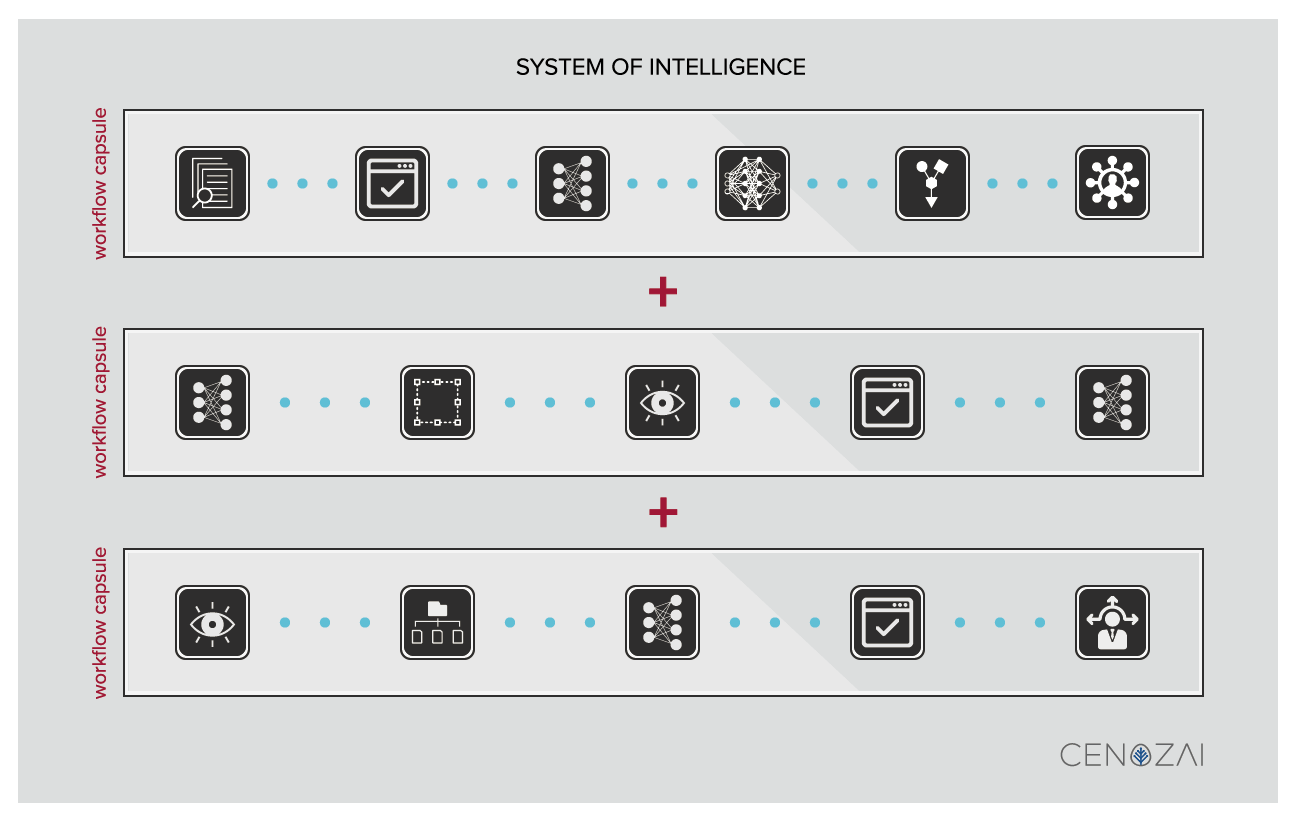
These systems are composed of modular “workflow capsules,” which can be stacked upon each other as needed. We’ll take a deeper look at why they’re designed this way through an example later on. First, let’s discuss another key element of an SOI: It allows human and machine to step through complicated processes together in a streamlined fashion. Why is it so important that humans and Artificial Intelligence work in tandem?
Irving Wladawsky-Berger sums it up nicely:
Most occupations involve a number of activities or tasks. Some of these activities are more susceptible to automation, while others require judgment, social skills and other hard-to-automate human capabilities. But just because some of the activities in a job have been automated, does not imply that the whole job has disappeared. To the contrary, automating parts of a job will often increase the productivity and quality of workers by complementing their skills with machines and computers, as well as enabling them to focus on aspects of the job that most need their attention.
What Machine Learning Can and Cannot Do →
For many people, working with content is a means to an end. For instance, if you’re a petrophysicist, your goal is to assess data to find zones of interest that may contain hydrocarbons. To do that, you’d need to search for—and through—documents and databases to find relevant information, use it to make predictions, and then judge those predictions to make good decisions. You could summarize that in an equation:
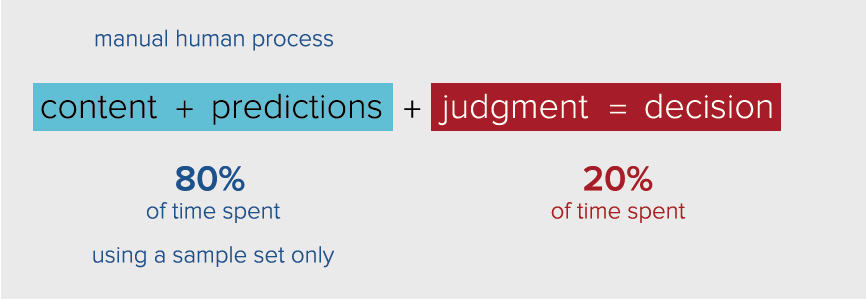
In a completely manual process, you may spend 80% of your time on tedious tasks, like sifting through content and generating predictions, leaving just 20% for exercising judgment. Additionally, given time constraints, you’d only be able to process a limited amount of data. You might miss a lot of relevant information.
AI, paired with knowledge graphs, dramatically increases the efficiency of accessing content and generating predictions. Because a machine’s computing power and ability to handle high dimensionality is so much greater than that of a human, it can also take into account all of your data, rather than just a subset. This leaves more time for judgment and decision-making—tasks where humans significantly outperform machines.
Keeping the Human-in-the-Loop
The human still has a role to play in the content and prediction piece, correcting content extraction and validating predictions. That’s because it’s rare for a machine learning algorithm to achieve 100% accuracy for any task. If it has, it’s probably overfitting to that task and cannot generalize when it encounters different data. So if an algorithm is performing at 94% accuracy, you can choose to correct errors to bring the results to 100%, or if 94% is good enough, leave things as they are. Bringing the human into the loop gives your SMEs more control over the process.
Systems of Intelligence: A Use Case
Let’s step through a facility maintenance planning use case: Reducing unit maintenance cost by revamping maintenance setup. At a high level, you’d need to:
- Create a hierarchical asset register
- Generate Bill of Materials for all assets
- Analyze equipment criticality; failure modes and effects; backlogs and costs; and run root cause analysis
- Establish the new maintenance plan and generate task sheets
Now let’s take a closer look at how to achieve the above. In a traditional workflow, you’d need to assemble a large team comprising process engineers, maintenance specialists, and so forth, to manually execute something like the following.
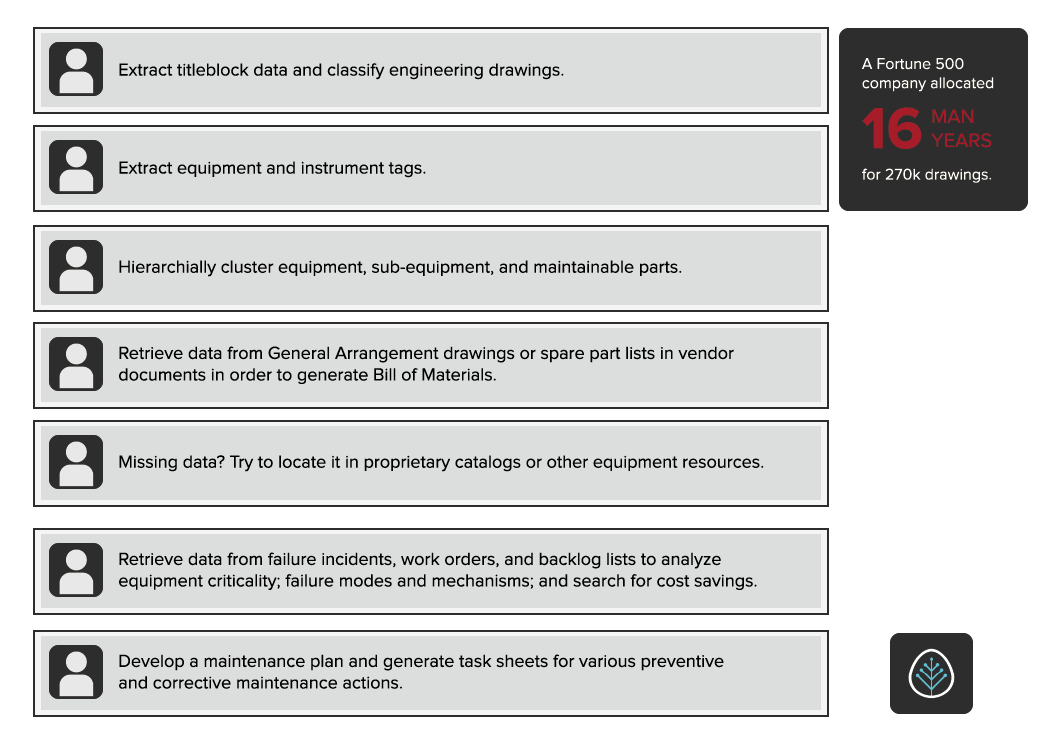
That’s an awful lot of coordination and labour required to accomplish a monumental task. You might even decide that it’s not worth the effort.
By combining domain expertise, machine learning, and knowledge graphs into a single System of Intelligence, a potentially intractable task becomes feasible.
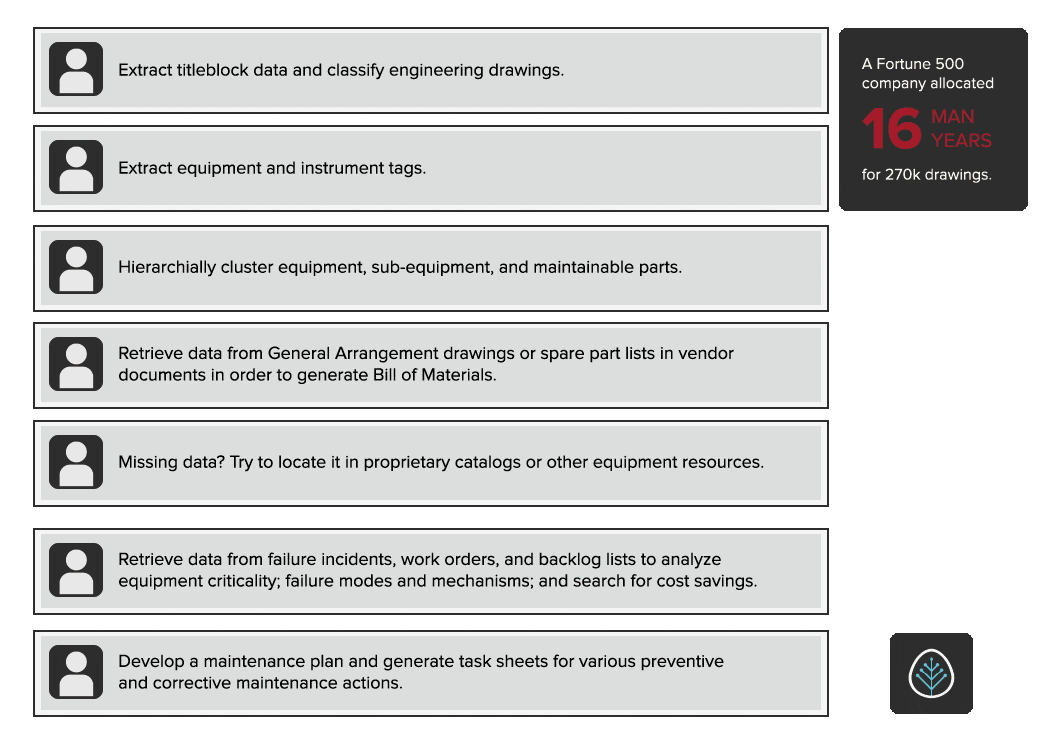
However, depending on where your company is in its digitalization process, you may not need all of these steps. Since Systems of Intelligence are modular and stackable, you have the flexibility to turn off particular modules in a workflow capsule if you don’t need them—perhaps it’s unnecessary to extract title block data and classify engineering drawings because you already have that information in your database.
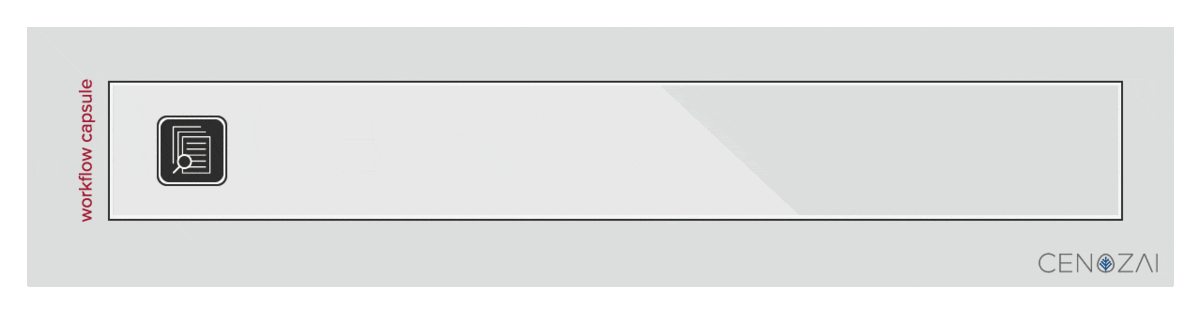
Similarly, you can also stack workflow capsules, seamlessly going from the legacy data extraction workflow described above to a workflow that handles predictive maintenance tasks, for example.
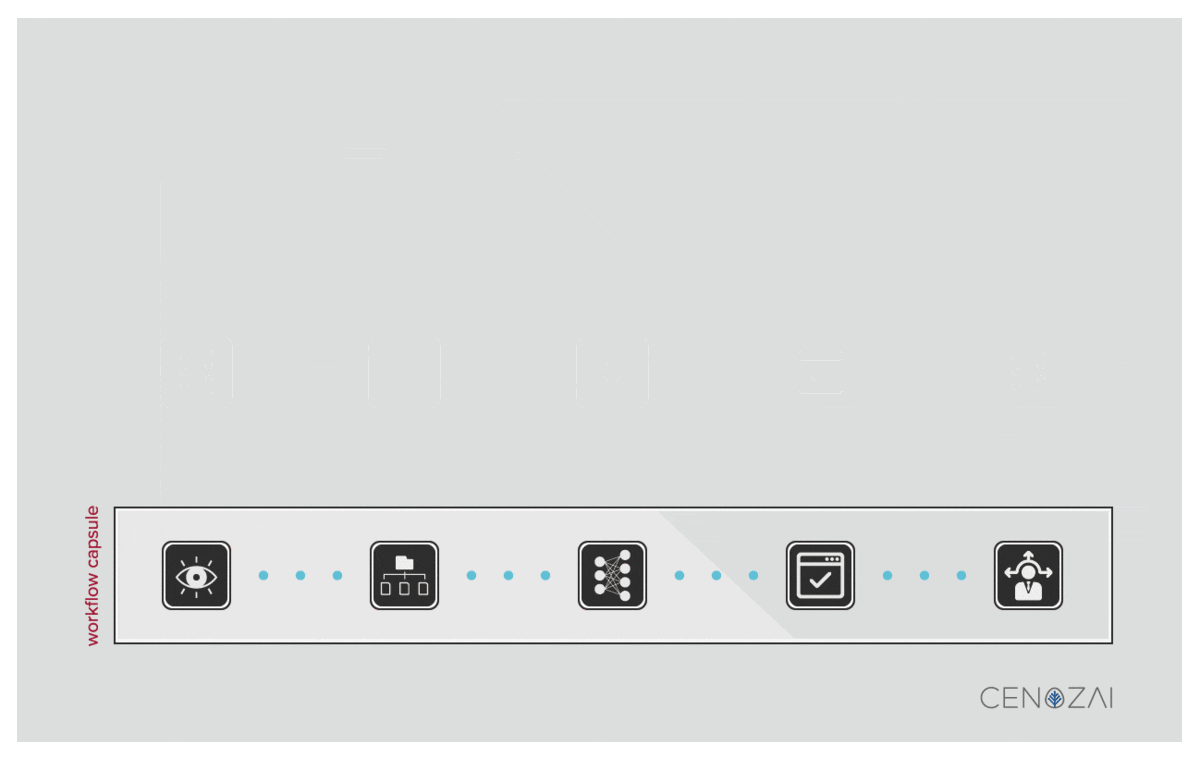
Your Subject Matter Experts (SMEs) shouldn’t spend their time searching through thousands of documents to retrieve information. Machine learning and knowledge graphs help with that. They also shouldn’t need to spend time figuring out which technology to use and how to make sure data from one feeds well into another. By consolidating multiple, curated tools and workflows in a single environment, your SMEs can focus on their core function: Making informed decisions.
Is a System of Intelligence a good fit for you?
There are a few questions you need to ask to determine whether a system of intelligence makes sense for your project.
- Does achieving your end goal entail navigating complex workflows?
The core purpose of an SOI is to streamline this complexity into an optimized system so that you and your team don’t have to worry about building the process yourselves. If, however, there’s no big process around what you need to do, or you’re running small, quick data science experiments, you’re better suited to checking out standalone machine learning tools. - Does the workflow require SMEs from several teams or departments?
If yes, then an SOI is a great fit. It can be difficult to find and assemble all the right people—especially experts—to complete the task. SOIs are designed to help someone with less experience bridge the gap. - Does your workflow require you to search and process reams of unstructured content?
Machine learning and knowledge graphs provide significant return on investment when it comes to dealing with one of the biggest challenges businesses face today: Unstructured content. Using a combination of computer vision, natural language, and graph techniques, an SOI can help your team parse through millions of documents that an expert system simply could not handle. It allows you to spend less time processing data, and more time on making better informed judgments to improve the quality of decisions. This not only improves productivity, but boosts morale by reducing tedious tasks.
Build your SOI team
Choosing the right people to operate your SOI is critical to your project’s success. Since an SOI typically covers a broad discipline scope, some modules can—and probably should—be operated by a consultant. Your SOI provider should be able to recommend the optimal course of action for you. Regardless, the general question to ask is:
Is this SOI element a core function of my business?
For example, extracting equipment and instrument tags from engineering drawings is a one-off task that’s a means to an end for oil company engineers working on maintenance planning. You’re probably better off engaging a consultant to help operate that module of the SOI. Cenozai’s platform allows you to select your own consultant, or use one of our specialist partners who have been trained to operate our software.
For the elements of a workflow that are core functions of your business, just deploy the discipline sets that you normally would. You can do that because SOIs do not require machine learning, data science, or graph expertise.
Additionally, since SOIs implement domain-specific machine learning and graph technology, they inherently embed and make predictions off of the knowledge of experts in the field. That allows you to assemble a team with less seniority for each discipline. You can then keep your scarce experienced resources focused on other tasks that require their attention.
Recommended Posts
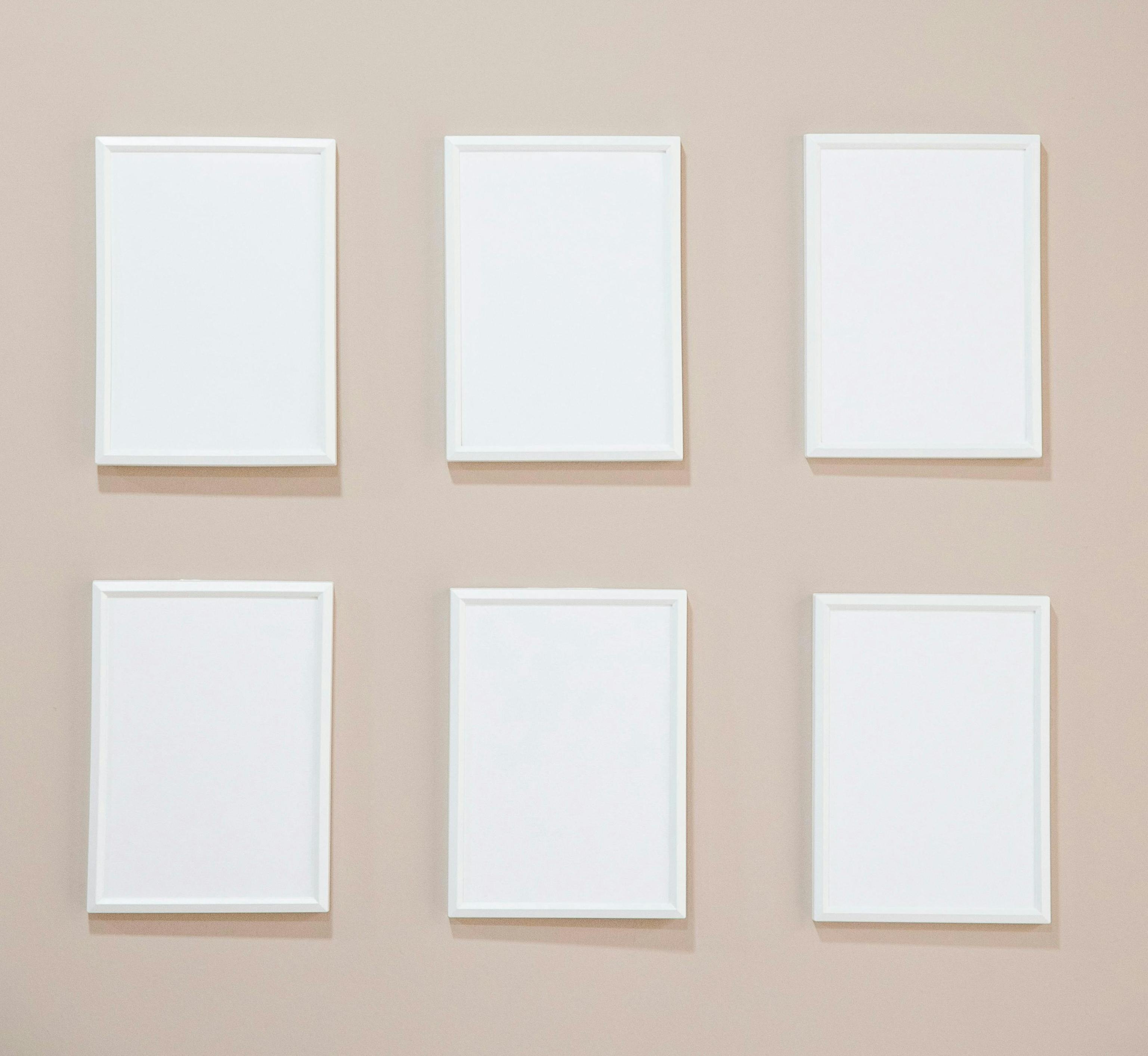
8/15/2024
A new era of enterprise search for facilities
(Part II): Data preparation
Before implementing new search technology, data cleanup and preparation is critical. Here's what you need to do.
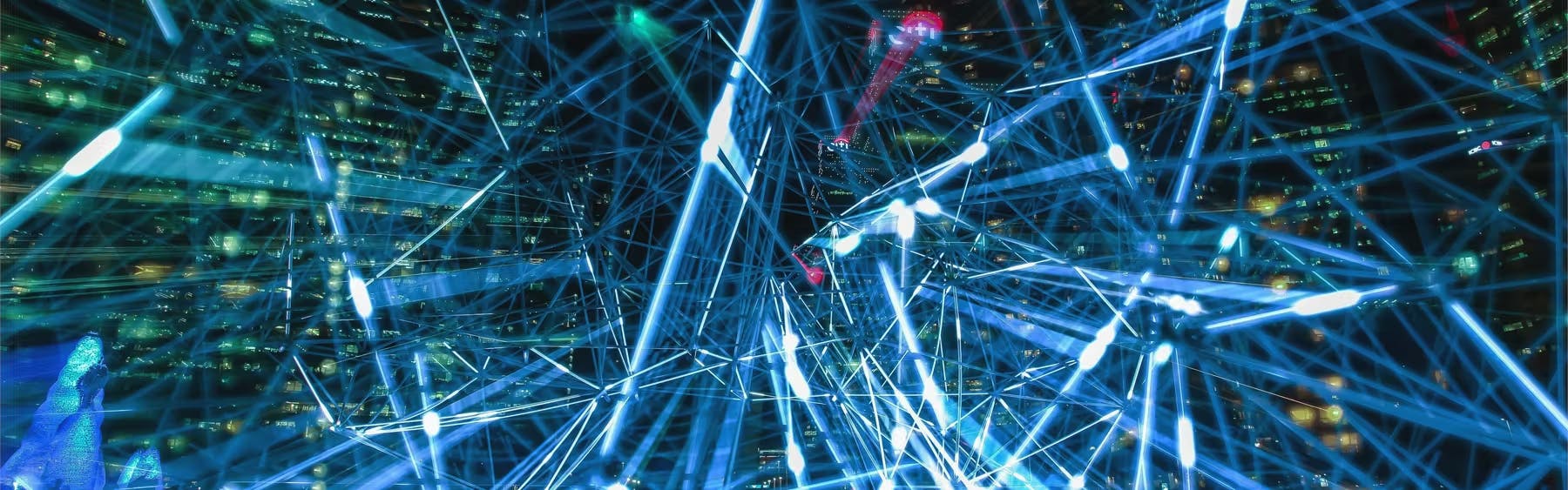
8/1/2024
A new era of enterprise search for facilities
Enterprise search is changing for facility operators—out with the file hierarchies and in with the information networks.
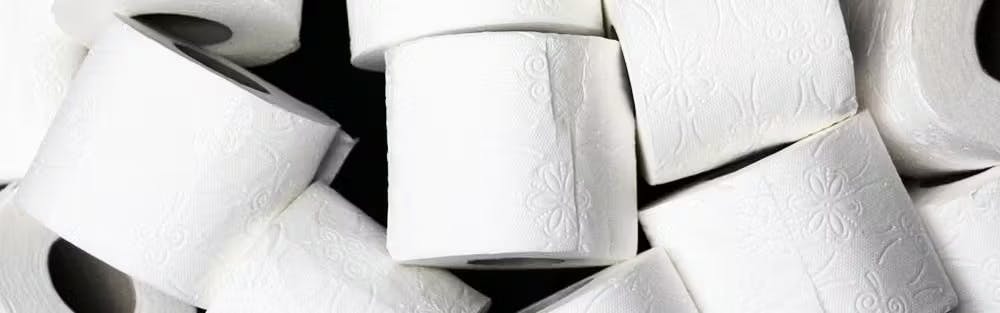
8/9/2022
Data hoarding is a major risk for
facility operators
Data hoarding is widespread, and has a major impact on business performance. What’s the problem, why does it happen, and how can you fix it?